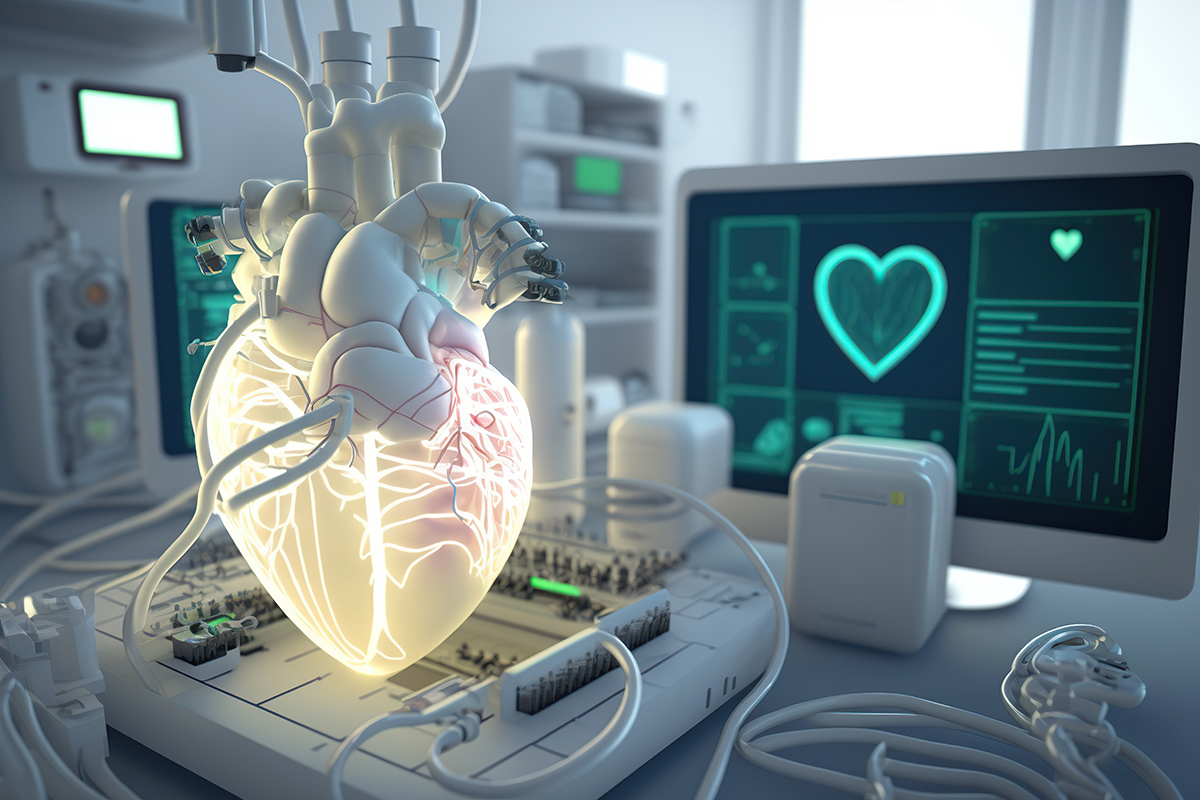
As the information age subsides, enabling the uprise of the artificial intelligence (AI) age; expectations and responsibilities need to be redefined. We spoke with key leaders in the WesternU Community regarding the current and future use of AI in medical education.
Steven Snyder, PT, DPT, CSCS
Assistant Dean, Technology and Innovation/Associate Professor
Department of Physical Therapy Education
Tim Wood, DHSc, PA-C
Associate Vice President, Associate Professor
Center for Excellence in Teaching and Learning (CETL)
Tracy Mendolia-Moore, MA EdManager of Educational 3D Technology, Technology Innovations,
Center for Excellence in Teaching and Learning (CETL)
Q. What is AI in medical education?
Steven Snyder: AI in medical education is a broad concept. There’s a lot of different ways it can be used. One way that I’ve seen it used in some of my early experience with AI is in adaptive learning. I’ve seen AI being used to help students really focus on their deficits, to build them up and get their deficits relatively close to their strengths to focus on where students need more time and coming up with different ways to understand that content. Another way I’ve seen AI in medical education is seeing students using it as a study tool. In addition, AI can be used by medical educators themselves to really help reduce certain workloads to make things easier or to facilitate certain processes within the educator’s role.
Q. Talk about AI tools and technology throughout medical history? What are the common uses and applications of AI?
Tim Wood: There is AI, but then there’s something different called generative AI. Chat GPT came out about a year ago and people really hadn’t heard of generative AI. In medicine (or actually “medicine in the clinic”), we’ve had AI for a long time within our electronic health records (EHR). Within the EHR, the AI would accumulate the responses based upon the subjective answers in the soap note that the clinician received from the patient. If there were certain constellations of answers checked off in the subjective portion of the soap note, as well as in the objective portion of the soap note that the clinician had observed, the AI (or the database) would check to see if there was a specific constellation of checkmarks, then it is most likely one of these 5 diseases. The EHR would generate for you what your most likely differential diagnoses were and then you as the human element in the room, would be able to verify more facts to determine the primary diagnosis. When we get into medical education, it was always a question of when will AI pop up?
Steven Snyder: Some of the common applications and uses of artificial intelligence within medical education are students using it to go deeper to understand concepts a little bit more. Students can take a concept that they learn in class that maybe they’re not caught up to speed on, and they can ask generative AI like Chat GPT. “Hey? Can you explain this like, I’m a high school student?” or “can you explain this like I’m 5 years old.” So, you kind of simplify that concept, get that basic understanding, and then build back up their understanding to that medical level. It can also be used to practice certain subjective examination skills. We need to learn how to talk to our patients and how to understand certain responses and with generative AI like Chat GPT, we can set up prompts. An AI chat bot can act as if they have a certain condition or if prompted right and given the right constraints can respond with appropriate subjective cues that might help a student get a little bit deeper into a condition or diseases, so we can use it to even help students practice subjective exams in a much more controlled way. By using a chat bot, we can practice those skills without affecting live patients.
Q. How are DO students using or could be using AI? Why is it important?
Tracy Mendolia-Moore: There is a Flashcard Plugin. I know many of the COMP & COMP-Northwest students use, a flashcard program called Anki, and there’s an Anki plugin if you use GPT 4, which is the paid version of ChatGPT; 3.5 is the free version. The paid version allows you to have access to over a thousand Custom GPTs, or plugins, which enhance the AI experience with specific customizations and can connect AI to 3rd party applications such as Anki. This allows students to easily create robust flashcards using AI, and then seamlessly add them to the Anki app to study for the course. The Anki GPT is just one example, but there are some good use cases for it.
Tim Wood: To expand, students will do a test review by themselves, or they’ll remember a question they got wrong, and they’re like, so what is the difference between conjugated versus unconjugated bilirubin? And why did it cause jaundice in this patient, but not in this other patient. ChatGPT is great to ask that question while the student is thinking about it and studying because they’re probably not going to write an email to the professor. They may be embarrassed to even ask that level of a question, but it’s something that was more basic that they forgot about and needed to be reminded of. It’s the personal tutor aspect that’s powerful for students. A lot of faculty miss the vision of that and they immediately go to, “Oh, well, I’m asking them to complete an assignment, and they’re putting in the prompt, and they’re getting the full assignment out and not doing the critical thinking on it.” We must help faculty understand that it is a productivity tool, and it can help students, it doesn’t just hurt student learning, but can enhance it.
Q. What are your thoughts on policies, plagiarism, and AI?
Tim Wood: Once the word on ChatGPT and generative AI got out, many faculty became very alarmed, because they feel that this generative AI tool is something that is going to have the students plagiarizing all the time. They’re thinking that the baseline level of knowledge doesn’t need to be learned because the students can access it through a generative AI model. I feel that this is what the real conversation about AI and medical education is right now.
Steve Snyder: Policies will need to be put in place to help limit that.
Q. Speak about data, privacy and security.
Tracy Mendolia-Moore: Data, privacy and security is a big topic. Many folks don’t read the end user license agreement; it’s just it’s “too long, didn’t read” (TLDR), right? But, folks should know that the information you’re entering is, in some cases, being saved by that generative AI system. What are they going to do with it? How will ChatGPT use the information you just entered into their chatbot? What will Microsoft Bing or Google Bard do with all these queries? Oh, and Heaven forbid…Never put FERPA or HIPAA or PII information into any of these chatbots. That is a huge, patient/student privacy issue. As you’re engaging with all these generative AI systems, use them with caution, and be very careful with the content you’re putting in.
Steven Snyder: Given that many artificial intelligence platforms receive a lot of that information and use it to further develop that intelligence through machine learning, anything you put in can be used for further iterations. We really can’t be putting in identifying information for patients or students. Everyone really needs to be aware of these security issues. Anytime you put something out into something that uses machine learning or artificial intelligence, it goes out and can be used further. So, making sure that we’re taking student or patient identifying information out of anything we put in is critical. If we’re going to use this technology which has amazing capabilities, we must be responsible with it, and be aware of the risks.
Q. What are some biases in AI?
Tim Wood: I believe there are biases in AI. Many of these large language models (LLM) were created and programmed using existing data lakes/pools/warehouses that can contain data that is biased. This goes back several years to when facial recognition software was first created. It was biased against people of color. The AI/facial recognition software was having a harder time because the algorithms were set up based upon traditional white faces as opposed to including a significant number of other ethnicities. These other ethnicities were not as easily recognizable (or were flagged) at a higher percentage and level because there was bias present within the algorithm. I think any of the algorithms moving forward regardless of the large language, there’s still more data on more affluent people, People with higher socioeconomic status could afford computers 12 years ago more easily than those of lower socioeconomic status. With this increased access to the digital domain (which is where the data is coming from) more data was being collected for this cohort. As an example, with increased digital access, more Google searches were performed and recorded on this group of people – and that’s where a lot of LLM data is coming from. Whereas those in lower socioeconomic neighborhoods didn’t have connectivity, couldn’t buy a computer, and/or didn’t have Wi-fi, so there’s less data about them. The large language models are trained on this more affluent population, because that data is available due to this cohort of people having earlier access to digital resources and data that could be recorded and attributed to them. (Bacchini & Ludovica, 2019; Cavazos, et al., 2020)
Q. What are some common misunderstandings about AI?
Steven Snyder: I think one of the common misunderstandings is thinking that all AI platforms are going to be created the same, or that all are going to have access to that same information which helped develop them. Certain artificial intelligence platforms have limited data sets that they were trained on, and others are more open to larger data sets on the internet.
Tim Wood: Another common misunderstanding is that AI can perform critical thinking, but it doesn’t. It’s looking at everyone else’s product of critical thinking and then it’s summarizing it. People think that it’s an entity that can think; it is not. You, as the user, are still responsible for verifying the output from the generative AI agent. You can give it whatever prompts that you want. However, based upon some common misconceptions that are reflected in the data (and that the large language model has accumulated and assembled), the user will receive faulty output. Therefore, it’s still your ultimate responsibility to verify that the output is correct and accurate. AI is NOT alive. As most of us have heard, hallucinations happen because it uses predictive text, and it is not able to critically think on its own.
Steven Snyder: I really don’t think AI will ever replace the human aspects of medicine because it’s a machine and it must be taught. There’s still mistakes that are made and it’s not able to do the clinical reasoning, or the critical thinking that’s really needed for effective patient care and effective management. And it’s never going to have the same level of empathy, human touch that an actual person will.
Q. How will AI shape the future of Medical Education? Where does Artificial Intelligence go from here?
Tracy Mendolia-Moore: There’s amazing things that are happening right now that Google is actually creating. In December, Google announced Med-PaLM, which is an AI designed specifically for medical research. Microsoft is also coming out with some things and recently partnered with Epic to expand AI within Epic’s EHR system. These are two of the largest tech software companies that are treading this path for us to follow. I think we’re going to see a lot of change over the next year and it’s just going to get even more intertwined into everything that we do and everything that we see. Everything that we touch now is going to have some AI component, whether or not you know it. That’s why it’s going to be really important that our students, our faculty, our staff, everyone is embracing it and can see the potential of what it can do.
Steven Snyder: AI is going to dramatically change the face of medical education. It’s going to help enable more people to be successful in medical school, it’s going to create new pathways for students to either have adaptive tutoring or adaptive learning. It’s going to allow students to ask questions or have things explained differently without utilizing as much class time or as much office time. There are more opportunities for practice with a standardized AI patient that’s able to respond in a much more natural way but be consistent within the responses appropriate to their condition. It offers a lot more opportunities for learning in different styles. It’s going to be able to provide faster feedback to students, more justification on correct versus incorrect answers as well. In addition, as it advances and pairs with other technologies like augmented reality, there’s going to be immersive learning. When they’re in a treatment room or an operating room, they can be familiar with the environment, but rather than just having a static field around them, things are able to adapt and change on the fly, because of that, AI will be able to kind of guess what’s the next appropriate response to what that student just did.
Q. How is AI changing Medical Practice?
Tim Wood: Based upon what you click in your soap note, it’s going to give you the most likely diagnosis and/or treatment. It is then up to the clinician to use their human brain to interpret and interpolate what the AI is suggesting. This is the time for the human element to make sure that the picture that was in the documented soap note versus what the patient looks like sitting in front of them matches. It is the clinician’s job to make the information consistent to get the best diagnosis and the best medical treatment that’s evidence-based to improve patient outcomes.
Tracy Mendolia-Moore: It’s changing every day. Recently OpenAI came out with image recognition within ChatGPT and I just saw an article this morning where an individual uploaded a picture of ringworm and asked ChatGPT to identify it. The AI not only accurately identified the ringworm, but it also provided treatment suggestions for the condition. AI can not only interpret text, but now it can also interpret images, X-rays, medical scans, MRIs, and so on. Every day it is changing, and getting more advanced.
Steven Snyder: Some of these generative AI technologies have helped reduce the writing load. There are certain ways in which it may eventually be used in helping speed up documentation. Even patient intake forms may be sped up or facilitated with the use of AI. Instead of a patient having that form where they’re checking boxes, and they’re not quite sure what the form is asking or what a response means. If that can be facilitated through an AI chat bot, where it seems like a person is asking patients these questions, or they could ask for clarification before checking a box and then what the provider gets on the other end is kind of our standard form. That would be helpful, increase patient comfort, and may improve accuracy with the practitioner, double checking those facts as well. So even image generation through AI, if I need to describe something I may be able to utilize. An AI image generator to create something rather than tell me to draw stick figures.
Q. What will the role be for health care providers that are working in an AI world?
Tracy Mendolia-Moore: I was sitting through a webinar about large language models specific to healthcare, and one of the things that they focused on was how AI could offset a lot of the administrative paperwork and processes, freeing up practitioners to spend more quality time with their patients. This means that instead of divided attention and multitasking between chatting with the patient and typing away in the EHR system, with AI as an admin, providers can focus on the person in front of them and can provide a more personalized and humanistic level of care. The AI can free them up to do what they do best – caring for patients, making those critical decisions, and adding that personal touch that no machine can. So, AI will be able to help lift some of those mundane and repetitive admin duties.
Q. What does this mean for the future?
Tracy Mendolia-Moore: Generative AI has really become an education disruptor, so there are a lot of theories out there. But, with AI, we really don’t know what the future is going to look like in the next few years, so how do we best prepare our students for a future that doesn’t yet exist? Talking about it is the first step. We must engage our university community, learn from each other, and continue to innovate. It’s truly an exciting time to be in education, as we will be the ones to help create that future.
Tim Wood: We need to train healthcare practitioners that know how to use AI to its fullest advantage. Our health professions students are going to be the ones that come out ahead in the next 2, 3 or so years than the people that don’t know how to use AI. The question becomes how we use AI thoughtfully within the curriculum as opposed to assuming everyone is going to plagiarize. It’s sitting down and looking at a different way of teaching and using AI to help do that, but also a different way of assessing because that’s where everybody is losing their minds right now. You can still do assessment, and especially at a higher-level assessment, but having your students use AI as a tool to get there. It’s just going to take a little more elbow grease from the faculty as well.
References
- Bacchini, F., & Lorusso, L. (2019). Race, again: how face recognition technology reinforces racial discrimination. Journal of information, communication and ethics in society, 17(3), 321-335.
- Cavazos, J. G., Phillips, P. J., Castillo, C. D., & O’Toole, A. J. (2020). Accuracy comparison across face recognition algorithms: Where are we on measuring race bias?. IEEE transactions on biometrics, behavior, and identity science, 3(1), 101-111.